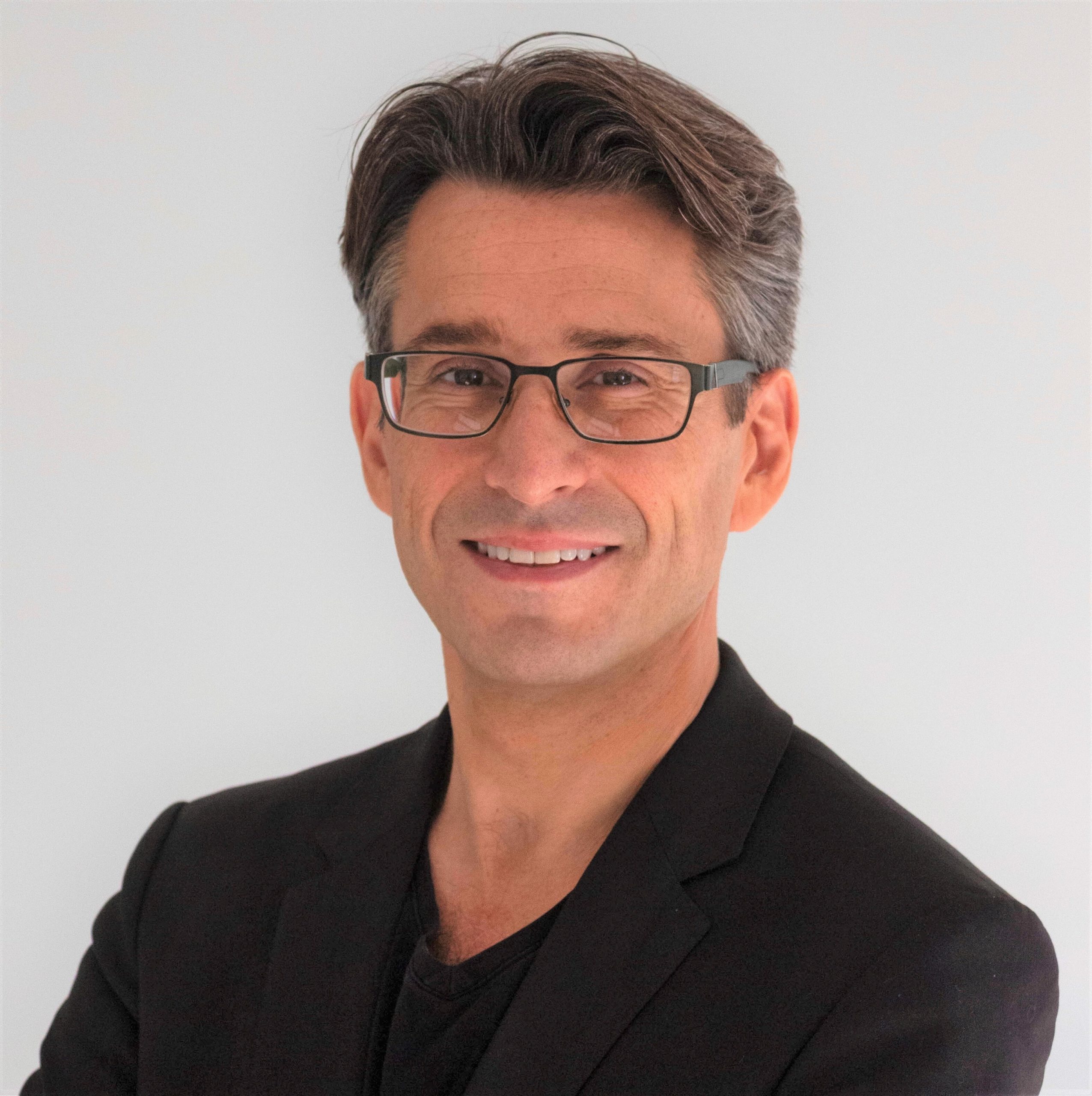
Dr Rowland Illing DM MRCS FRCR
Director & Chief Medical Officer, International Public Sector Health, Amazon Web Services and Honorary Associate Professor of Image-guided Intervention,
University College London
Artificial intelligence is nothing new. The term was used for the first time in 1956 by John McCarthy. However, AI development was slow due to a lack of computing power and availability of data. As computing power and data volumes have grown at exponential rates, AI adoption has followed.
For many years now, health information has been collected in a digital format, accounting for petabytes of data. However, this data has not been used to its full potential. This was primarily due to unstructured information consisting of different types of data: medical imaging, physician notes, genomic sequences, etc. There was no easy way to sort this data and extract valuable information from it. Artificial intelligence (AI) and machine learning (ML), including generative AI (GenAI), are now proving to be the solution to this problem.
AI enables automation in telehealth tools
The use of AI in healthcare is not a new phenomenon, with some of the earliest work dating back to the MYCIN project in the 1970s. However, the Covid-19 pandemic acted as a catalyst to accelerate adoption and drive innovation. Automation is inextricably associated with AI, and telehealth was the first area in the health sector automated by AI. This includes the process of setting up appointments or talking to a virtual nurse assistant through chatbots (chat robots) or voicebots (voice robots).
Utilising chatbots for efficient communication
Chatbots allow patients to talk to a computer using natural language. AI-powered voice recognition and voice transcription turn what you speak into text. Natural language processing (NLP) converts this text into commands understandable for computers, which they can process. With voice generation (text-to-speech), they can also answer questions. As a result, patients no longer have to queue for an hour to set up a doctor’s appointment. With AI-powered voicebots, setting up appointments is now instant.
There are more functions that are harnessing voicebots. Patients can even get initial advice from a ‘computer-powered doctor.’ A voicebot can ask them about their symptoms and, in many cases, the computer can run a triage and suggest a treatment or — with more serious issues — suggest visiting a doctor.
Examples of ML detecting Covid-19 and cancer
During the pandemic, scientists at Massachusetts Institute of Technology (MIT) devised a machine learning system that was able to analyse specific features in a patient’s cough to determine if they were an asymptomatic carrier of Covid-19.
Another example is image recognition (also known as computer vision), which is possible with deep learning (a subset of ML). Computers can now analyse radiology images to identify a narrow range of specific conditions, such as blood clots in the lung arteries (pulmonary embolism) or cancers with up to 99% accuracy. While they can do it thousands of times faster than a human, these results require ‘expert in the loop’ clinical validation from trained radiologists.
AI enables democratisation of access to care and early detection
AI also helps enhance the operation of ultrasound devices, meaning less training is needed, by aiding in interpreting captured images and ensuring more consistent measurements. All this significantly improves the early detection of potential diseases, which has the potential to save thousands of lives, especially in areas where specialist skills are less available.
Patients can even harness this technology on their own. SkinVision, a Netherlands-based company, developed a solution for detecting skin cancer at an early stage. By taking a photo of a concerning skin lesion, patients can utilise a deep learning-powered solution to determine whether it is benign or potentially malignant. In such cases, patients are directed to seek medical attention for further evaluation.
Through process automation in the provider
workflow, specialists will be able to focus less
on paperwork and more time on direct patient care.
Application across various healthcare fields
AI is used across medical imaging as well as other data types, including text, audio and genomics. This drives automation of clinical processes (for instance, diagnosis) and administrative tasks (such as scheduling).
According to the World Health Organization (WHO), there is a one in a million chance of a person being injured while travelling by aircraft. In comparison, there is a 1 in 300 chance of an individual being harmed throughout the patient journey. Prior research has shown that up to 50% of all medical errors in primary care are due to administrative reasons. The global shortage of medical professionals further compounds these problems. The WHO also estimates a projected shortfall of 10 million health workers by 2030, mostly in low and lower-middle-income countries. This makes it increasingly challenging to provide care to everyone in need.
It is AI in administrative tasks that is likely to have the most immediate impact on patients, as it can make every step of their journey smarter, not only scheduling through chat and voicebots. By analysing patient admissions, discharges and hospital capacity in real time, providers can concentrate care on the most critically ill patients. Systems are being built that detect patient deterioration and automate the follow-up of urgent or unexpected findings.
There is also a great potential benefit to care providers themselves. Through process automation in the provider workflow, specialists will be able to focus less on paperwork and more time on direct patient care. Enabling care providers to work ‘at the top of their license improves efficiency of the system and personal job satisfaction.
Advanced processing of vast data with generative AI
The latest turning point in AI is the development of generative AI (GenAI), which is a subset of deep learning. Traditional machine learning models required labelled data to train their neural networks, which makes them specific to the area of interest (therefore they are ‘narrow,’ usually answering specific questions). In comparison, GenAI is trained on vast troves of unlabeled data to develop foundation models (FM).
These FMs look at relationships and associations between many different parameters, which can uncover previously unknown connections. They can be used to extract and summarise data and even generate new content, depending on the data they were trained on. The impact on healthcare will be tremendous — whether collating patient data to make it easier for doctors to make diagnoses, understanding patient risk from numerous different variables (their genetic make-up, as well as environmental exposure), designing new treatments (such as the Moderna Covid vaccine) and even making doctors’ letters more patient-friendly to read.
How GenAI can impact populations
GenAI will not only impact the care of individuals; it can also analyse population-scale data. Genomics England is using machine learning to integrate and explore connections between genetic sequences, imaging and clinical history in the UK as part of their Cancer 2.0 programme for multi-modal data. Also, pharmaceutical companies are working with GenAI to identify similar patients (cohorts) who may be suitable for therapies, clinical trial enrolment and prediction of therapy response.
Who can make use of GenAI?
Until recently, AI was used only by machine learning experts who were able to write code and build pipelines from scratch. One benefit cloud computing brings is that specific ‘services’ can be incorporated into applications that enable data scientists (rather than ML experts) to automate some of the data processing that is required.
In the case of Amazon Web Services (AWS), the ‘Sagemaker Studio’ accelerates time to build new AI applications, and there are specific tools (Sagemaker Jump Start and Bedrock) that can accelerate the utilisation of a range of Foundation Models from Amazon.com and third-party providers. In the future, there will be hundreds, if not thousands, of FMs; the data the model is based on will indicate what kind of indication it is used for. Therefore, democratising access to the widest possible selection of FMs is imperative to provide choice for users.
AI aids professionals and helps cut costs
Importantly, AI is not going to replace human physicians or nurses. It simply helps them to automate tasks and run many of them hundreds of times faster than before. It also helps significantly reduce costs, especially administrative costs, which are now estimated to be around one-third of all spending on health in the US — twice as much as the US spends on caring for cardiovascular disease and three times what it spends on cancer care.